Unlocking the Power of Image Labeling in Modern Business
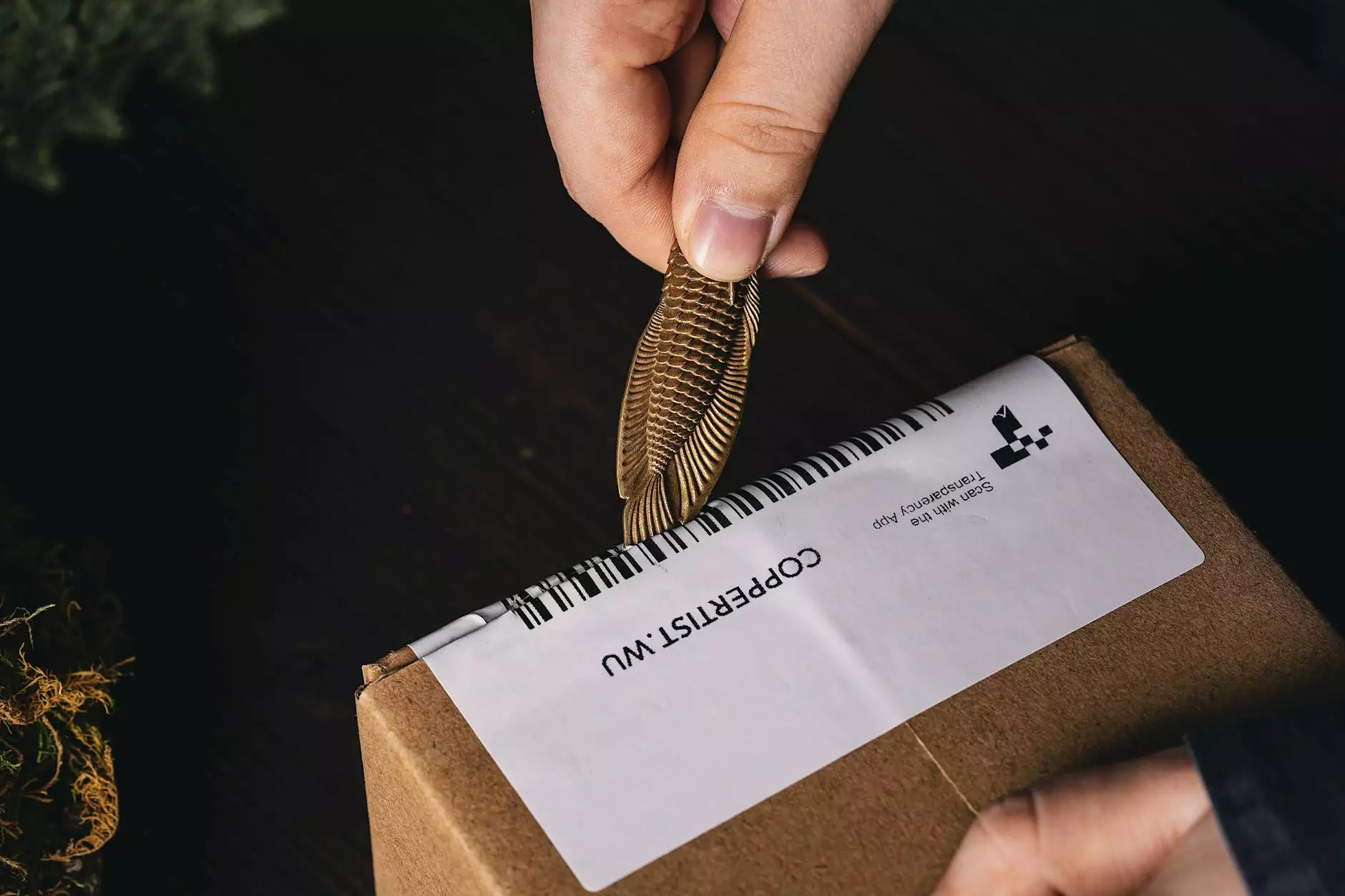
In today's digitally driven world, the need for high-quality data to train artificial intelligence (AI) models has skyrocketed. One of the critical components of data preparation is image labeling. Whether you're involved in machine learning or computer vision, having a robust data annotation process is essential for achieving superior accuracy and performance in AI applications.
What is Image Labeling?
Image labeling is the process of identifying and tagging objects within images. This not only aids in understanding the context of the images but also plays a pivotal role in training AI models. By providing structured information, labeled datasets enable machine learning algorithms to learn patterns and make predictions accurately.
Importance of Image Labeling in Various Industries
Image labeling is not just a technical task; it's a transformational process across various industries. Let's explore some of them:
1. Healthcare
In the healthcare sector, accurate image labeling is crucial for developing AI models that assist in diagnostics. For example, labeling medical images can help in:
- Detecting abnormalities: AI models trained with labeled images can recognize patterns associated with diseases.
- Predictive analysis: Labeled data can streamline patient care through predictive modeling.
2. Automotive
The automotive industry relies heavily on computer vision for autonomous driving technology. Image labeling helps in:
- Object recognition: Identifying pedestrians, vehicles, and obstacles in real-time.
- Route mapping: Enhancing navigation systems with accurately labeled imagery.
3. Retail
In retail, image labeling enhances customer experiences and operational efficiency by:
- Product categorization: Automatically labeling product images for smoother inventory management.
- Visual search: Allowing customers to search for products using images rather than text.
The Process of Image Labeling
Understanding the image labeling process is essential for businesses looking to implement effective data annotation strategies. The steps include:
Step 1: Collecting Images
Gather a diverse set of images that adequately represent the data you want your AI model to understand. Diversity is key to preventing bias.
Step 2: Choosing the Right Tools
Select specialized data annotation tools. A robust platform like KeyLabs.ai offers several features that cater to your labeling needs with ease and efficiency.
Step 3: Manual vs. Automated Labeling
Decide between manual labeling (performed by humans) and automated labeling (performed by AI). While manual labeling ensures accuracy, automated systems can speed up the process significantly.
Step 4: Quality Assurance
After labeling, it's imperative to have a quality assurance process in place. This may involve double-checking labels and using statistical methods to ensure labeling consistency.
Step 5: Finalizing Datasets
Once the images are accurately labeled and verified, compile them into datasets ready for training your AI models.
Key Features of an Effective Image Labeling Tool
Choosing the right data annotation tool for image labeling can significantly impact your project’s success. Here are some key features to look for:
1. User-Friendly Interface
A tool that is easy to navigate helps reduce the learning curve for new users and increases overall productivity.
2. Customizability
Customization options allow teams to adapt the tool's functionalities to meet specific project requirements, including labeling styles and workflows.
3. Collaboration Features
Given that many teams work on projects, having built-in collaboration tools ensures seamless communication and efficiency among team members.
4. Integration Capabilities
The ability to integrate with existing systems or AI frameworks enhances workflow and minimizes the disruption of established processes.
5. Scalability
As your business and data volumes grow, your labeling tool should easily scale to accommodate increasing demands.
Challenges in Image Labeling
Despite its importance, image labeling comes with its own set of challenges:
1. Time-Consuming Process
Labeling large datasets can be tedious and time-consuming, especially if done manually.
2. Ensuring Accuracy
A high level of accuracy is crucial. Mislabeling can lead to poor model performance, necessitating rigorous review processes.
3. Handling Diverse Data
Diverse datasets can introduce complexity, requiring nuanced labeling that captures the variances in imagery.
Leveraging Technology for Improved Image Labeling
Advancements in technology are reshaping how image labeling is performed. Some innovative approaches include:
1. Machine Learning-assisted Labeling
Using machine learning models to suggest labels can significantly reduce the time and effort needed for manual labeling. These models can learn from previously labeled data to assist in future annotations.
2. Crowd-sourcing
Utilizing a crowd-sourcing approach for data labeling can harness a large workforce, increasing efficiency and enabling faster turnaround times.
3. Active Learning
This technique involves training models to identify which samples need labeling, thereby optimizing the labeling process and focusing efforts where they yield the most value.
Conclusion: The Future of Image Labeling
Image labeling is a vital cog in the wheel of AI-driven innovation. Businesses across various sectors are realizing the potential of accurate data annotation to enhance their operations. By investing in efficient image labeling tools like those offered by KeyLabs.ai, organizations can gain a competitive edge in their respective industries. The fusion of cutting-edge technology, skilled labor, and rigorous quality control will pave the way for unparalleled advancements in AI.
In summary, the future of image labeling is bright. As industries continue to integrate AI into their workflows, the demand for precise and efficient image labeling will only continue to grow, making it a cornerstone of a successful digital transformation strategy.